When making an image with light waves or sound waves, you can't capture details smaller than the wavelength unless you use some clever tricks. These tricks often require great expense and computational power, but a new technique using machine learning is much simpler and better suited to situations where modifying the object to be imaged is not possible [1]. The method captures details as small as 1/30 of the wavelength employed and should be useful in areas such as biomedical imaging and nondestructive materials testing.
- Forkless installation uses a single 1/4-20 (M6) cap head screw. The internally slotted base allows for versatile positioning and solid clamping. Using a 3/16 in. Ball tip Allen wrench, one can access the 1/4-20 (M6) mounting screw via the patent-pending angled access slot in the pedestal.
- Ford's biggest struggle will be getting buyers to pay attention to the Focus instead of defaulting to a more popular Corolla, i30, Mazda 3 or Golf. It sits closer to those top sellers than ever.
The conventional limitation on resolution for a given wavelength is called the diffraction limit, and it arises because of an important difference between the so-called near-field and far-field waves either emitted by or reflected from an object. The far-field waves, which travel long distances, contain information about features larger than a wavelength. The near-field waves carry information on finer scales but die away before traveling very far and therefore don't reach lenses that might gather the waves to produce an image.
To overcome this problem, researchers in the past have placed some secondary objects near the object to be imaged. These secondary objects have the correct size and shape to serve as resonant cavities that amplify the near-field waves, re-radiating the information on the object's finer details in the form of nondecaying waves. However, the creation of a final image from this re-radiated information has required sophisticated technology and intensive processing, and such methods acquire images only slowly. Other methods require less processing but need radiating elements to be placed inside the object to be imaged [2] and so don't allow for a noninvasive technique that would be useful for medical imaging. Romain Fleury and Bakhtiyar Orazbayev of the Swiss Federal Institute of Technology in Lausanne (EPFL) have now developed an imaging system that uses external, secondary resonators but needs far less computing power than previous methods.
The researchers first showed that the idea works with model calculations and then demonstrated it in experiments using 1.3-meter-wavelength sound waves. They used 64 small speakers arranged in an 8×8 array roughly 25×25 cm in size. Rather than aim these speakers at an object to be imaged, the team treated them like a set of pixels whose individual 'bright' or 'dark' states could be produced by controlling each speaker's loudness. They broadcast the audio equivalent of an image that played the role of the sound signal produced in a conventional imaging system.
For the images, the researchers selected from a database of 70,000 handwritten variations on the numbers 0 through 9. The digits contained some features that were about 30 times smaller than the diffraction limit. For the secondary elements, the team used thirty-nine 10-cm-diameter plastic spheres, each with a small hole in its surface, which allowed sound waves to resonate inside. These spheres were collected in a mesh bag in front of the speaker array.
For a series of randomly chosen digits, Fleury and Orazbayev recorded the amplitude and phase of the arriving sound with microphones at four locations a few meters away. Two different machine-learning algorithms used these raw data to learn—one trying to reconstruct the pattern as accurately as possible and the other trying to classify it as one of the ten digits. The algorithms first trained on a set of known signals and then were tested with new signals not found in the training set.
Working first in the absence of the intermediate spheres, Fleury and Orazbayev found that the algorithms performed poorly. However, in the presence of the spheres, the algorithm aiming to reconstruct the images did so effectively, and the one aiming to classify the digits achieved an accuracy of 79.4%. 'We were actually surprised by how well the method worked,' says Fleury.
In medical imaging, the researchers suggest, the algorithms would be trained not on numbers but on a database of known biological structures. The algorithms could then recognize similar structures that they hadn't seen before and would require little time or processing power to do so.
'This is an important and beautiful piece of work,' says optics and acoustics expert Sébastian Guenneau of Imperial College London and the French National Center for Scientific Research (CNRS) in Paris. 'Essentially it shows that you can beat constraints imposed by the laws of physics by using some machine-learning algorithms.' He believes the technique will likely have diverse uses in areas ranging from cancer detection to seismic monitoring to acoustic tomography in early pregnancy tests.
Dual Focus: Dual focus has dual speed focus knobs that offer both faster and finer focus adjustment; Rotating Ring: Rotating Ring allows you to rotate the scope around tripod supporting ring into the most comfortable and convenient angle for observation.
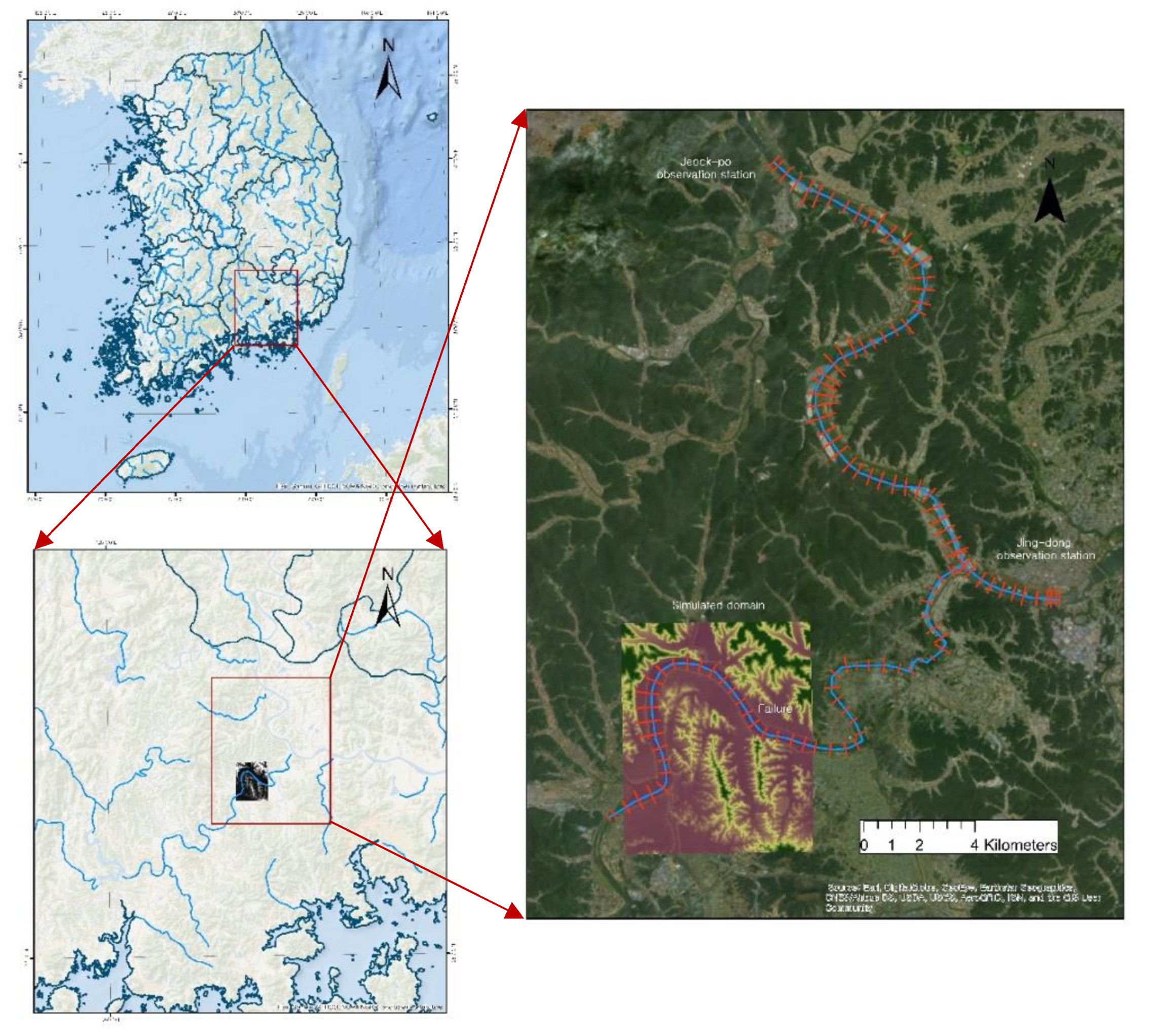
This research is published in Physical Review X.
Mechrunner 1 0. –Mark Buchanan
Mark Buchanan is a freelance science writer who splits his time between Abergavenny, UK, and Notre Dame de Courson, France.
References
- B. Orazbayev and R. Fleury, 'Far-field subwavelength acoustic imaging by deep learning,' Phys. Rev. X10, 031029 (2020).
- P. Hoyer et al., 'Breaking the diffraction limit of light-sheet fluorescence microscopy by RESOLFT,' Proc. Natl. Acad. Sci. U.S.A113, 3442 (2016).
Far-Field Subwavelength Acoustic Imaging by Deep Learning
Finer Focus 1 3 Sezon 1
Bakhtiyar Orazbayev and Romain Fleury
Published August 7, 2020 Adobe acrobat dc v20 006 20034.
Subject Areas
Related Articles
AcousticsDetecting Acoustic Blackbody Radiation
A nanomechanical resonator detects and isolates acoustic waves from a remote thermal source. Read More »
AcousticsMaking Materials Mimic Each Other
Finer Focus 1 3 Sezon 3
A novel framework for controlling many-body systems with external fields shows how two distinct materials could be made to mimic each other or form more exotic materials. Read More »
Condensed Matter PhysicsBoxes Go Topological for Sound
In a chain of 3D printed boxes, only the first transmits sound waves with certain frequencies, evidence of a topological edge state. Read More »